LuminLab
Pioneering a digital building energy consulting service to accelerate the energy efficiency upgrade of buildings in pursuit of a sustainable green transition at the urban scale
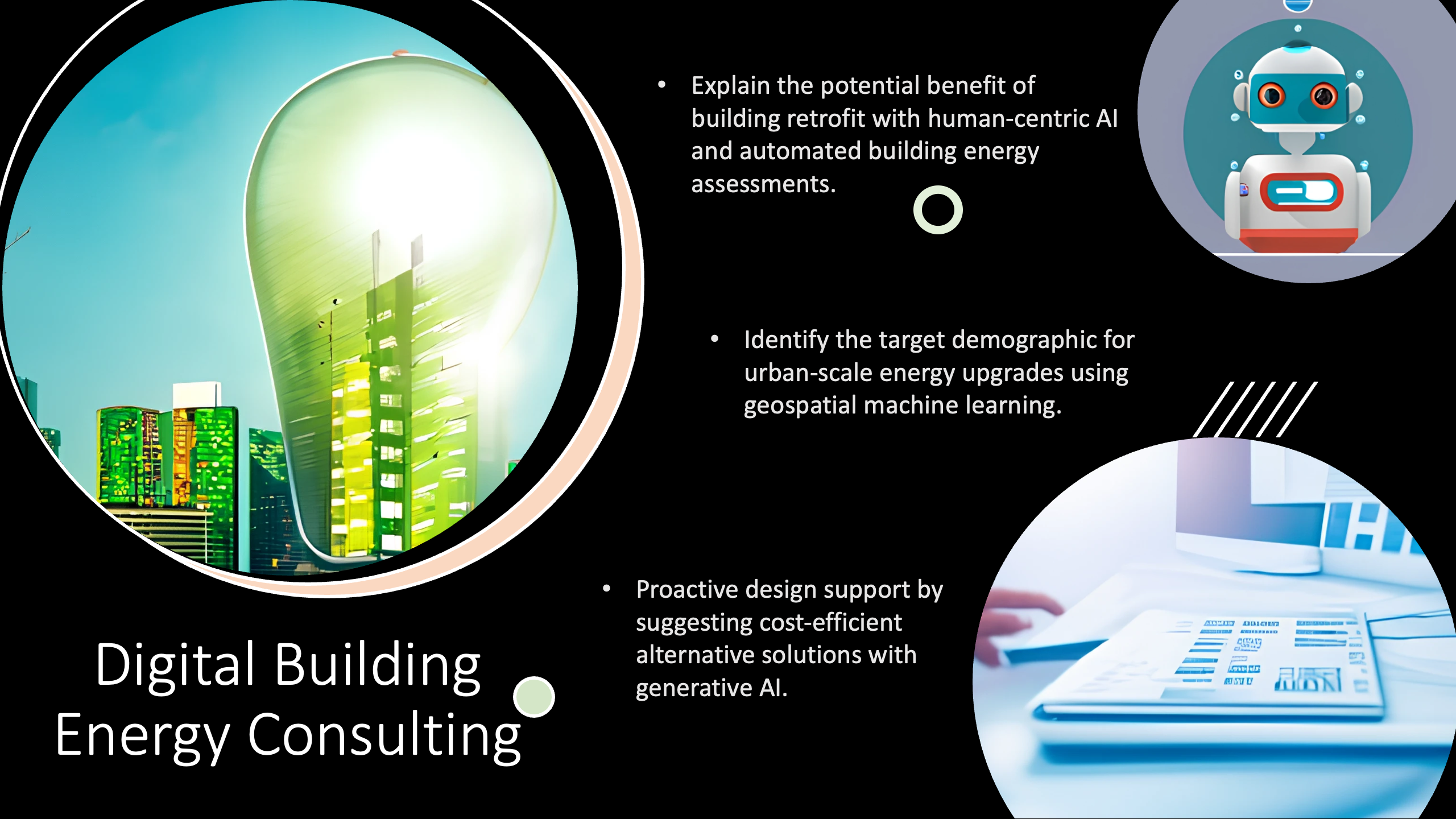
In Brief
- Challenge: Future Digital Challenge
- Challenge Type: National Challenge Fund
- Status: Active
The Challenge
Buildings are the centre of human activity in cities. Improving building energy efficiency, especially for existing structures, is key to combating climate change for many EU cities. Urban building retrofitting faces significant challenges in practice but without strategic intervention in the retrofitting problem, at urban scale, our society won’t be able to transition to carbon-neutral by 2050 as expected in the Paris Agreement. Upgrading urban building efficiency will contribute to the welfare of the whole urban population, and especially vulnerable members of society and those at risk of fuel poverty.
The Solution
We will use large-scale AI models to tackle these challenges, for both commercial and residential building energy upgrades, from three aspects of sustainability: economic, social and engineering. We aim to advance machine learning techniques by enabling model reuse via knowledge distillation, which can effectively mitigate performance drops due to data-acquisition difficulties. By socioeconomic analysis, we seek to reshape our understanding of energy poverty and develop impactful strategies that can influence local and regional energy use and planning. By exploring approaches to support generative design, we aim to enable rapid trial and testing in the planning workflow, and thereby help urban planners, building owners and designers identify viable and more cost-efficient solutions to improve building efficiency at an early stage. Empowered by human-centric AI technology, our service explores realistic solutions by integrating technology with data intelligence and analytics of the urban economy to simplify the customer journey and foster collaboration among public-private partnerships.
The Team
- Team Lead: Dr Qian Xiao, ADAPT Centre
- Team Co-Lead: Dr Kevin Credit, Maynooth University
Societal Impact Champion
- Dr Stephen Brennan, Digital Hub